Pfizer Uses Model-Based Drug Development to Help Reduce Phase II Attrition Rates
“Pfizer is integrating modeling, simulation, and statistical analysis throughout drug discovery and development. This approach helps reduce phase II attrition by guiding the selection of the best biological pathway, target, molecule, dosing regimen, and patient population.”
Challenge
Solution
Results
- Insight into the drug-body interaction deepened
- Costly laboratory resource spending and clinical trials avoided
- Research productivity increased
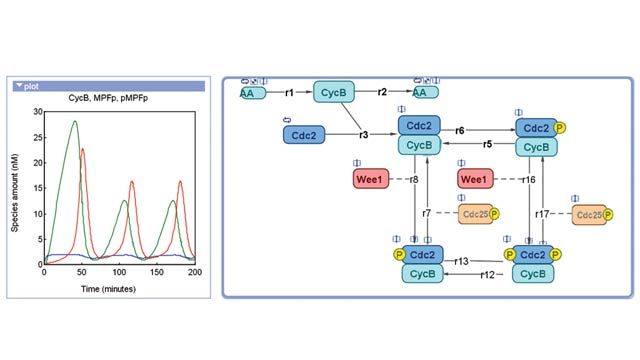
By the time a new drug reaches phase II clinical trials, the pharmaceutical company has invested millions of dollars and years of research and development. If late-stage trials show that the drug is insufficiently effective or causes unacceptable side effects, much of that investment is wasted. This phase II attrition poses serious challenges for pharmaceutical companies, not only for financial reasons, but also because it drains development resources away from successful new treatments.
Pfizer researchers use computational tools such as MATLAB® and SimBiology® to support model-based drug development and help reduce phase II attrition. “A growing database of published biological studies provides snapshots of the enormously complex systems that make up the human body,” says Dr. Neil Benson, associate research fellow in the department of Pharmacokinetics, Dynamics and Metabolism (PDM) at Pfizer. “SimBiology enables us to construct sophisticated models of biological systems based on available research data. By simulating these systems we can better understand their kinetics and dynamics and then use that understanding to focus our research on the most promising biological targets.”
Challenge
Pfizer is committed to model-based drug development. “Phase II attrition needs to be tackled at the root causes—right from the selection of the biological target in early discovery,” says Dr. Piet van der Graaf, senior director, Pharmacometrics and Clinical Pharmacology at Pfizer. “We wanted to apply modeling and simulation in the earlier phases of drug research and bridge the gap between systems biology and pharmacokinetic-pharmacodynamic (PK/PD) modeling approaches.”
The text-based interfaces of some modeling software packages can make it difficult to construct and share increasingly sophisticated models. “We had to write all the equations by hand. As our models got bigger, there were many more equations to type in, and constructing them and ensuring consistency of the units became very laborious,” says Benson. “In addition, when we needed to communicate our work with other researchers, the long list of equations was not easy for them to understand, particularly if they were not expert modelers.”
Projects typically require significant preclinical laboratory investment to build confidence in an approach and subsequent clinical trials to validate hypotheses. To avoid spending millions of dollars and years of research effort on projects or approaches that cannot succeed, Pfizer wanted to be able to identify and terminate them earlier.
Solution
Pfizer has started to develop an integrated Systems Pharmacology approach in which researchers use tools such as MATLAB and SimBiology to model, simulate, and analyze biological systems in the earliest stages of discovery and throughout development.
Researchers construct Systems Pharmacology models to answer questions about a given pathway, such as which receptors are likely to be the best targets and what concentration of drug compound is needed to achieve the required inhibition.
Sometimes the group bases the initial model on a published Systems Biology Markup Language (SBML) model, imported directly into SimBiology and other software tools.
They next perform sensitivity analysis to identify and rank the most important targets in the model, using simulation events to model the introduction of a particular drug into the system.
They visualize results by reviewing diagnostic plots, including time course plots with and without the drug present.
Using visual interfaces, they share the model, the plots, and other simulation results with the project team for the drug, who provide parameter estimates or other input to further refine the model.
On one project, the team explored the effectiveness of using small interfering RNA (siRNA) to inhibit the IKK enzyme in the NF-kB pathway. After constructing and simulating a model with 26 species and 64 parameters, they found that the level of inhibition siRNA provided was not sufficient to achieve the needed result. Subsequent experimental results were consistent with this conclusion.
Pfizer is currently assembling a library of Systems Pharmacology model components that can be reused for different projects to further accelerate analysis using PK and systems biology models.
Results
Insight into the drug-body interaction deepened. “I’m not a mathematician, but with SimBiology I’ve constructed models with up to 400 ordinary differential equations,” says Benson. “SimBiology let me create more complex models than I ever had before, and provided deeper insight into how they worked by enabling analysis of their kinetics and dynamics.”
Costly laboratory resource spending and clinical trials avoided. “On multiple projects, including the one focused on siRNA and the NF-kB pathway, results from Systems Pharmacology have demonstrated that a particular drug would not work and ultimately the data generated were consistent with this,” notes Benson. “In the case of the siRNA project, the modeling could have been carried out prior to engaging any laboratory resource.”
Research productivity increased. “During drug discovery, lots of experiments can be performed,” notes Benson. “We use Systems Pharmacology results to focus these efforts and propose optimal experiments. We can narrow the experiments we perform, focusing on the most promising biological targets.”